Exploring Alternative Solutions: The Role of RAG in Legal AI Tools
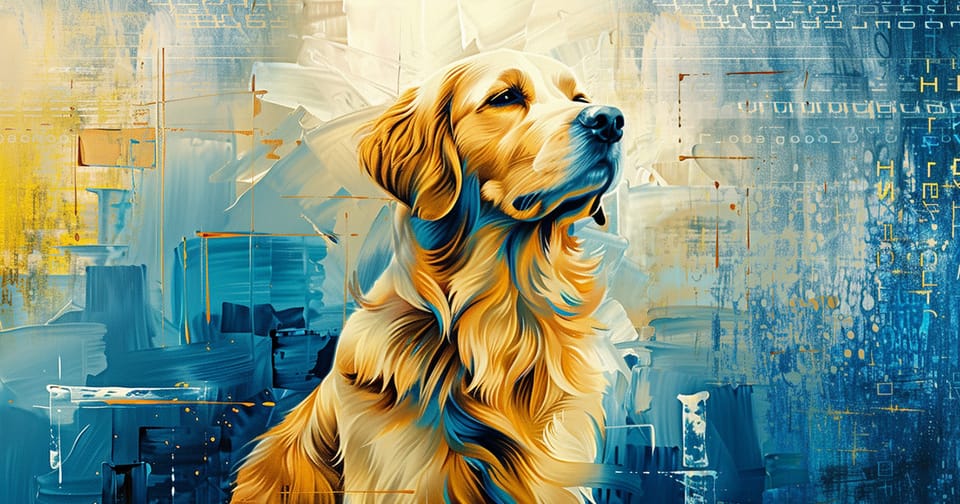
Editor’s note: This article is the third and final in a three-part series in which we explore and evaluate the use of Retrieval-Augmented Generation (RAG) for AI-powered legal tools. Although this technology can increase the accuracy of generative AI outputs, recent studies have illuminated its shortcomings. Mindful of the importance of accuracy in the legal industry, Prevail takes a unique approach to building our AI tools using alternate methods.
In our first article in this series, we covered the recent Stanford Study on hallucinations in AI Legal tools and the industry backlash that resulted from it. Common-use AI tools, like Chat GPT and Claude, along with RAG-based AI tools by Thomson Reuters and LexisNexis, were tested on how accurately the tools responded to legal queries. The results of the study were troubling. All of the AI tools tested in the study hallucinated to some degree, causing concern throughout the legal industry.
Our second article illustrated that RAG is a vector-based AI search method designed to ground AI responses in factual information. RAG is, essentially, an AI tool that adds an extra step to its generated responses by first retrieving information from relevant sources. We also discussed in the article that RAG isn’t foolproof; the accuracy of its responses relies heavily on the quality of the sources it retrieves information from. Even when the sources are correct, RAG can misinterpret information and doesn’t handle nuance well.
The study and its results revealed significant issues with RAG-based AI tools, especially in legal contexts. AI isn’t perfect. However, we also felt it necessary to discuss how Prevail’s AI-enhanced platform differs from RAG and common-use AI tools. Prevail has taken a distinct approach to building AI tools, which we believe mitigates many of the challenges highlighted in the study. This series aims to shed light on these differences and offer a clearer understanding of how AI can be used responsibly and efficiently in the legal industry.
Alternative Approaches to RAG in Legal Tech
When it comes to AI-powered legal tools, RAG is often seen as the go-to method, but it isn’t the only option available. Before we discuss the Prevail difference, let’s explore some other alternatives to RAG and how they compare.
Fine-Tuning:
This method involves training the model on specific datasets to specialize in certain domains. Rather than retrieving external information, the model learns from a narrow, curated dataset. This can lead to more efficient and coherent responses since the model is specialized. However, it lacks flexibility. Any new information requires retraining, making it less adaptable for fast-evolving fields like law.
In-Context Learning:
Here, relevant information is directly included in the prompt, so the model doesn’t need to retrieve it from external sources. This method is simple to implement and avoids the complexity of retrieval systems. The downside is the limited amount of information that can fit within the model’s context window, meaning it may struggle with large or complex datasets.
Knowledge-Grounded Models:
These models are pre-trained on large knowledge bases, integrating information during training rather than retrieving it later. The advantage is that these models can access a broad knowledge base at inference time without reviewing external sources. The trade-off is that their knowledge becomes fixed after training, making it difficult to update with new information.
Hybrid Approaches:
Some models combine techniques, such as fine-tuning with retrieval. This hybrid method can capitalize on the strengths of multiple approaches, offering flexibility and efficiency. However, these systems tend to be more complex to implement and maintain, requiring careful balancing of the methods used.
External Tool Use:
This method involves the model using external tools or an Application Programming Interface (API) to retrieve up-to-date information rather than pulling from preset data and generating text. This is particularly useful for specialized or real-time data. However, the effectiveness of this method depends on the availability and reliability of the external services the model relies on.
Prompt Engineering Techniques:
This approach involves crafting sophisticated prompt structures to guide the model’s output without relying on external retrieval. It can be highly effective for specific tasks, but scalability is an issue for more complex or variable use cases, where it may struggle to adapt.
Each of these alternatives has its strengths and weaknesses compared to RAG. The best choice depends on the specific needs of the task, available resources, and desired outcomes. While RAG remains a popular method, exploring these alternatives opens the door to potentially more efficient or specialized solutions.
The Prevail Difference
Prevail’s implementation of AI takes a unique approach to legal tech by moving away from traditional RAG models. Unlike RAG, which typically relies on vector-based search to retrieve results similar to a user’s query, Prevail’s system doesn’t simply search and return information. Instead, it goes further by reformatting the results to match the user’s query with greater precision. This allows Prevail’s AI to find relevant information and transform it to be even more applicable to the user’s specific needs, creating a tailored and accurate response.
One of the key distinctions is that our system doesn’t retrieve data based on the user’s query. Instead, it examines every piece of data against the predominant question being asked, minimizing the chances of errors or hallucinations. By focusing on specific use cases, Prevail can drill down into highly targeted questions, ensuring that the tool is much less likely to generate irrelevant or misleading information. This is in stark contrast to RAG models, which often place too much emphasis on the specific wording of the user’s query instead of the intention behind the query, a practice that can lead to misinterpretations and inaccuracies.
Unlike many emerging AI products, Prevail’s AI tools are far more than a simple GPT wrapper. Instead of relying on user queries to fetch answers, Prevail provides information and asks the system to categorize responses based on what is most pertinent. For example, if a user asked a RAG-based tool to “find all the dad jokes,” it might look for any reference to “dads” and “jokes,” missing the nuance that “dad jokes” are a specific genre of humor. Prevail’s AI is trained to recognize such subtleties, searching for meaning beyond the literal words of the query.
Another crucial difference lies in how our system handles context. As mentioned above, RAG tools can struggle with nuance and often take a query too literally. These tools search for similar words, not necessarily the contextual meaning behind the query, leading to inaccuracies in more complex searches. Prevail’s AI, on the other hand, is built to understand the broader context, recognizing connections and meanings even if the information isn’t a literal match. This ability to perform more granular and context-aware searches sets Prevail apart, ensuring legal professionals receive more accurate and meaningful results.
Human oversight is a critical factor that sets Prevail’s AI-enhanced platform apart from other AI tools. While our technology is designed to minimize errors and deliver accurate, context-aware results, it’s the careful involvement of our team that ensures these tools operate at their best. Our AI isn’t simply left to run on autopilot—it’s continuously monitored, refined, and evaluated by experienced professionals who understand the intricacies of legal work. This level of human oversight allows us to spot potential issues early and make adjustments that enhance the system’s accuracy and reliability. Ultimately, our AI is only as powerful as the people behind it, and this combination of advanced technology and expert guidance truly makes Prevail stand out.
The Path Forward for AI in Legal Tech
As the use of AI continues to grow in the legal industry, legal professionals must stay informed on how these tools are advancing. Ultimately, the future of AI in legal work will require balancing innovation with ethical responsibility. RAG offers clear benefits but has notable complications, so it’s essential to research, compare options, and choose the tool that best fits your organization’s needs.
Most importantly, AI tools require human oversight to catch errors, interpret nuances, and ensure that the technology is used ethically. While AI can significantly enhance legal processes, it doesn’t replace expert judgment. As advancements continue, the role of legal professionals will evolve, not to rely solely on these tools but to guide their application in ways that uphold the integrity and accuracy of the legal system. By staying engaged and critical of the technologies they adopt, legal teams can harness the power of AI while minimizing the risks it poses.
If your organization needs a secure, AI-enhanced platform with expert human oversight, look no further than Prevail. Book a demo today to learn more about how Prevail can help with your legal proceedings.