Breaking Down the Stanford Study: The Role of RAG in Legal AI Tools
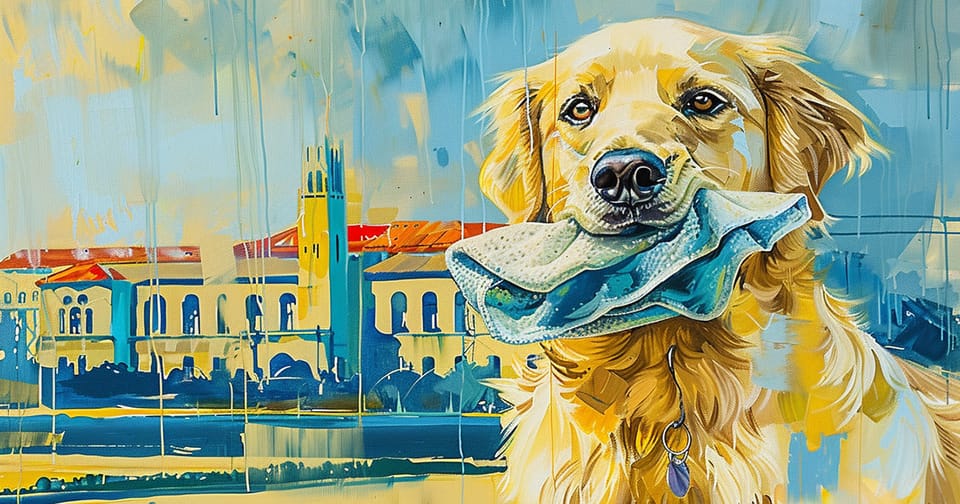
Editor’s note: This article is the first in a three-part series in which we explore and evaluate the use of Retrieval-Augmented Generation (RAG) for AI-powered legal tools. Although this technology can increase the accuracy of generative AI outputs, recent studies have illuminated its shortcomings. Mindful of the importance of accuracy in the legal industry, Prevail takes a unique approach to building our AI tools using alternate methods, which we’ll discuss later in this series.
Earlier this year, the Institute for Human-Centered AI (HAI), a research group at Stanford University, evaluated top generative artificial intelligence (AI) legal tools and found serious issues. Their published study revealed that some legal AI tools, trusted by many in the profession, often hallucinate—generating false or misleading information.
Researchers at HAI and Stanford’s RegLab began by testing the efficacy of large language models (LLMs) in legal contexts. The results? General-use chatbots like ChatGPT, Llama, and Claude hallucinated on legal queries between 58% and 80% of the time. The researchers then turned their attention to two giants in legal research: Thomson Reuters, creator of Westlaw AI-Assisted Research and Ask Practical Law AI, and LexisNexis, creator of Lexis+ AI.
HAI and RegLab researchers constructed pre-registered datasets of over 200 open-ended legal queries to test Thomson Reuters’s and LexisNexis’s tools in order to observe system performance. The tests included four types of queries: 1) general research questions, 2) jurisdiction or time-specific questions, 3) false premise questions, and 4) factual recall questions. According to HAI, “These questions are designed to reflect a wide range of query types and constitute a challenging real-world dataset of exactly the kinds of queries where legal research may be most needed.”
While Thomson Reuters and LexisNexis’s AI-powered research tools significantly reduced errors compared to general-use models, their hallucination rate was still alarmingly high. HAI and RegLab published these results, shocking the legal industry with their findings.
Industry Backlash to the Stanford Study
In the first version of the study, Thomson Reuters’s and LexisNexis’s AI tools had a similar hallucination rate, at 19% and 17%, respectively, which was concerning. Both companies tout a technology called Retrieval-Augmented Generation (RAG) as a key feature to ensure that generative AI responses from their legal tools are grounded in accurate, verifiable data.
Thomson Reuters and LexisNexis weren’t quick to accept the study's results. Both criticized the study’s methodology, with Thomson Reuters stating that Stanford didn’t use their tool for its intended purpose, thereby skewing the results. Stanford countered, claiming that Thomson Reuters denied their requests for access to the correct tool during the evaluation. Both LexisNexis and Thomson Reuters argued that the study didn’t account for real-world conditions in which their AI tools typically operate.
In response to the pushback, Stanford conducted a follow-up study, broadening its scope and refining its dataset to better reflect the tools’ practical applications. Despite the new parameters and both companies’ defense of RAG technology to bolster the accuracy of their tools, hallucinations persisted, often in critical ways. In the second version of the study, Thomson Reuters’ AI tool hallucinated in about 33% of cases, nearly twice as often as LexisNexis’s at 17%, exposing stark inconsistencies in how these systems handled complex legal information. These findings underscored the need for continued vigilance and innovation, particularly as AI plays a more prominent role in legal research.
What is RAG (Retrieval-Augmented Generation)?
RAG is a vector-based AI search method designed to boost accuracy by grounding generated responses in real-world data. Unlike common-use AI models, such as Claude, that rely entirely on pre-trained knowledge, RAG enhances the generation process by retrieving pertinent information from external databases. This allows the AI to draw on specific, up-to-date sources instead of depending solely on its internal memory. It’s a two-phase system that aims to reduce the risk of hallucinations.
The retrieval phase is the first step. Here, the AI processes the user’s query using natural language processing (NLP) techniques to search for relevant documents. It’s similar to a smart search engine, but instead of offering a list of results, the AI selects the most appropriate data from what it finds. Once the information is retrieved, the AI moves into the generation phase, using the gathered documents and large language models (LLMs) to craft a more informed, context-rich response. This process helps minimize the AI’s reliance on its internal data, making it less likely to hallucinate.
In legal contexts, RAG-based AI tools are often used to process vast amounts of information and quickly find relevant answers to the user’s query. For example, legal professionals may use RAG to expedite research, analyze various legal strategies, or for client consultation. RAG and AI tools, in general, have the potential to expedite and improve legal processes, but the concern about hallucinations is valid.
RAG is Not A Perfect Solution
LexisNexis and Thomson Reuters, among others, have embraced RAG as a way to decrease the hallucinations that often plague common-use AI models. These industry leaders recognized RAG’s potential early on and continued to invest in the technology even after the Stanford Study highlighted its shortcomings. LexisNexis has insisted that its users will see improvements "week over week" as the company continues to train its RAG-based AI system.
The ongoing commitment of LexisNexis and Thomson Reuters to RAG models underscores its significance in enhancing the accuracy and reliability of AI-powered legal research tools. However, the Stanford Study highlights the need for further research and development of AI tools, particularly for legal applications.
RAG models hallucinate far less than common-use AI models do, but they are not infallible. As Greg Lambert, Chief Knowledge Services Officer at Jackson Walker, recently told Artificial Lawyer, "RAG will help get the products part of the way there, but as this study clearly shows, the products can still bring back incorrect, but very convincing results, that can cause even good attorneys to fall for these hallucinations." According to Lambert, there’s an inherent “creativity problem” with RAG, but it’s not a glitch. Rather, this tendency to generate convincing but factually inaccurate responses is a feature of RAG that requires continued refinement and human oversight.
RAG, AI, and their use in legal contexts is a nuanced topic that deserves detailed attention, so it will be the focus of our next two articles in this series. Follow along and stay tuned for the next blog, in which we’ll examine RAG’s challenges and limitations related to legal tech.