Promises and Pitfalls: The Role of RAG in Legal AI Tools
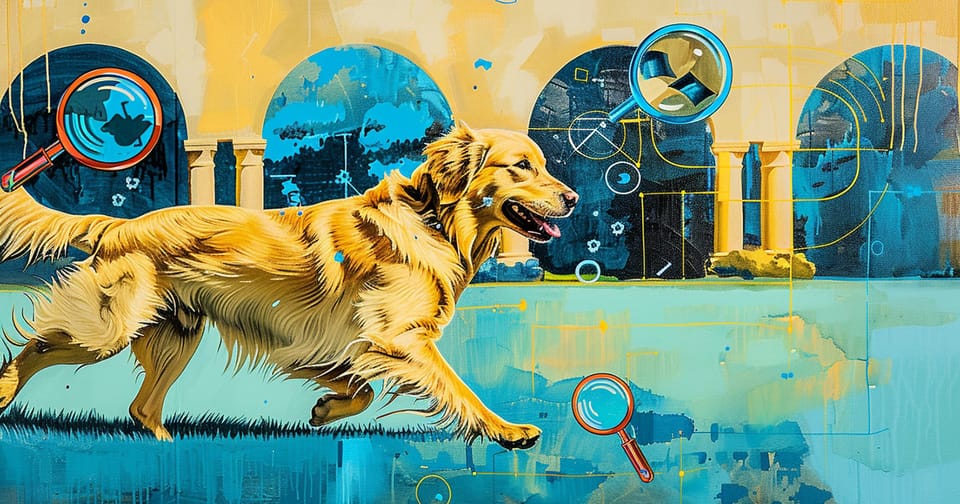
Editor’s note: This article is the second in a three-part series in which we explore and evaluate the use of Retrieval-Augmented Generation (RAG) for AI-powered legal tools. Although this technology can increase the accuracy of generative AI outputs, recent studies have illuminated its shortcomings. Mindful of the importance of accuracy in the legal industry, Prevail takes a unique approach to building our AI tools using alternate methods, which we’ll discuss later in this series.
Accuracy is everything in legal research, where even minor errors can have serious consequences. As discussed in our first article in this series, a recent Stanford study revealed that some legal artificial intelligence (AI) tools, trusted by many in the profession, often hallucinate—generating false or misleading information.
In the study by Stanford’s Institute for Human-Centered AI (HAI) and RegLab, researchers tested common-use AI tools, such as Chat GPT and Claude, and RAG-based AI tools in a legal context. The researchers found an alarming rate of hallucinations in both types of AI tools.
RAG-based AI tools, like those created by Thomson Reuters and LexisNexis for legal research, had lower rates of hallucination than the common-use models, but they still hallucinated, raising concern in the legal industry.
Although RAG can improve accuracy and many industries view it as the standard for error-free AI tools, RAG-based tools still make mistakes, which can be detrimental in legal contexts. This series aims to help legal professionals who use AI-enhanced tech to understand how RAG works, where it falls short, and what alternatives exist to ensure that the technology they rely on is dependable and precise.
Limitations and Challenges of RAG in Legal Contexts
Maximizing the potential and minimizing the risk of AI depends on understanding how it tends to succeed and fail, which can vary not only by technology but also by subject domain. Despite its potential, RAG is far from flawless, especially in legal contexts. As highlighted by the Stanford study, RAG depends heavily on the quality and relevance of the data it retrieves. This poses a particular problem for legal analysis—for one example, consider jurisdictional nuances. Because legal precedents vary across regions in ways that are too subtle for generic retrieval, RAG output may not reflect these differences accurately. Therefore, RAG-generated output requires additional scrutiny when handling multi-jurisdictional cases.
The risks aren’t confined to retrieval alone. After gathering information, the AI tool must generate a coherent response, and poorly processed data or irrelevant material can muddy the generation phase. Even with correct sources and queries, RAG sometimes retrieves or summarizes inaccurate information, leading to responses that miss the mark on legal questions.
Another hurdle? RAG-based AI models weren’t much better than common-use AI models at grasping the hierarchies of legal authority. A deep understanding of how courts interact across various regions is necessary to accurately assess which rulings apply to particular circumstances. The AI models tested in the study failed to account for these types of nuanced legal relationships, a mistake that could be made by any AI tool.
Even with direct access to case law, the tools don’t have legal background knowledge to draw from to give a correct answer to a query that requires jurisdictional expertise. While RAG can help in many cases, it clearly doesn’t eliminate the need for human oversight.
Ethical and Practical Considerations
The implications of RAG errors in high-stakes environments, like law, are impossible to ignore. Minor inaccuracies can have far-reaching consequences, from misinterpreted rulings to flawed legal arguments. Legal experts continue to debate the ethics of relying on AI tools that aren’t foolproof, highlighting the risks when errors slip through in complex cases.
Transparency is also essential for trust in AI systems. When legal professionals don’t understand how an AI tool operates, confidence in its outputs quickly erodes. The Stanford study underscored the importance of clarity in AI-assisted research—without it, the integrity of legal processes is at risk. AI tools must be open, auditable, and accountable to ensure reliability in legal work.
Additional ethical considerations:
- Informed consent: Legal professionals should know AI tools’ capabilities and limitations before using them. Without understanding how the technology works, there’s a risk of misusing it, which could lead to poor outcomes or ethical breaches.
- Bias and fairness: AI systems are only as objective as the data they’re trained on. If that data is biased, the AI’s outputs could reflect that bias, leading to unfair or skewed legal decisions. Ensuring fairness and neutrality in AI systems is critical for maintaining justice in legal contexts.
- Responsibility for errors: When AI tools make mistakes, who’s responsible? This is an ongoing ethical debate. In legal environments, the consequences of AI errors can be severe, yet the line of accountability isn’t always clear. Lawyers must be vigilant in reviewing AI-generated outputs to avoid liability.
These ethical considerations will become more pressing as AI influences legal work. Balancing innovation with responsibility is vital to the future of AI-assisted legal research.
RAG and Data Security
Data security is another aspect to consider when implementing AI-enhanced tools, especially within legal contexts. RAG-based AI tools can streamline processes, but there’s concern about their ability to protect confidential information. A recent report found that 80% of data experts believe AI increases data security challenges, which RAG-based tools don’t necessarily account for.
Legal work often requires access to sensitive information, and RAG systems pull data from external sources, which may raise confidentiality issues. Privacy is paramount when handling client data. Any vulnerability in the retrieval process could lead to breaches or expose protected information, which might result in financial repercussions. This possibility introduces a layer of risk that legal professionals must navigate carefully.
When RAG retrieves information from external databases, the security of that data largely depends on the protections implemented by a third-party source. If these sources lack proper encryption or access controls, sensitive information could be exposed during retrieval.
RAG also introduces the potential risk of data manipulation. If an external database is compromised, malicious actors could alter legal precedents or data, leading to AI-generated misinformation. Ensuring that retrieved data is accurate and reliable becomes a critical security concern.
Critical threats RAG poses to data security
- Data interception: Since RAG accesses external databases, the process of transmitting or retrieving data can be intercepted if encryption or secure channels aren’t adequately implemented.
- Inconsistent security standards: External data sources may not maintain the same rigorous security standards as the organization using RAG, leading to potential vulnerabilities.
- Data authenticity: There’s a risk of retrieving inaccurate, outdated, or manipulated data from insecure databases.
- Regulatory compliance: RAG could unintentionally pull data from non-compliant sources, resulting in breaches of laws like GDPR, HIPAA, or legal confidentiality regulations.
While RAG can improve accuracy and performance by integrating real-time data, it introduces security risks that require strict encryption protocols, verified data sources, and regular human audits to mitigate.
Ensuring Responsible Use of RAG and AI
RAG offers clear benefits but has notable complications, especially in legal tech. As technology advances, it’s crucial that legal professionals thoroughly vet their AI-powered tools. Understanding how RAG works and how well it might perform in various contexts and ensuring legal AI tools comply with security regulations are essential steps toward using AI responsibly. Additionally, legal teams must remain aware of the limitations of RAG and be prepared to intervene when the technology falls short.
No AI system is perfect, and relying solely on these tools without proper oversight can lead to costly mistakes. Combining an understanding of how RAG functions with human expertise empowers legal professionals to better navigate AI in law. Our final article in this series will cover alternatives to RAG-based systems and how Prevail is changing the game with our AI-enhanced platform and services.